Lexalytics Helps Technomic Catergorize Hundreds of Thousands of Food and Beverage Ingredients with 98% Efficiency Using AI
BACKGROUND
Technomic answers questions like, “What’s the next sriracha?” “Is the mocktail movement here to stay?” and “What’s the state of veganism?” Restaurants, supplier-manufacturers and retailers of all sizes rely on Technomic’s in-depth market intelligence to forecast and report on trends across the food and beverage industry.
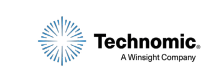
COMPANY: Technomic, Inc.
FOUNDED: 1966
EMPLOYEES: 200+
INDUSTRY: Food and Beverage Industry Client Research and Insights
We’ve been extremely impressed by the AI that Lexalytics has developed — it’s critical in helping us deliver the types of deep insights and trends reporting our customers expect And beyond Lexalytics’ incredible technology capabilities, the team’s been a wonderful and transparent partner. We’re looking forward to expanding the relationship.
— Adam Roberts, Director, Product Management, Technomic
CHALLENGE
To deliver these insights, Technomic collects sales data on hundreds of thousands of food and beverage ingredients from across the industry and adds hundreds of new ingredients every month. Technomic works with several data vendors to receive this data, and recently brought on another vendor to expand their knowledge base. Unfortunately, the new vendor categorized products differently than Technomic’s well-established hierarchy. For example, if an ingredient was labeled “Sauce Mix Alfredo Add Milk ZTF 4/1 Lb,” Technomic needed to bucket that ingredient under the category “Alfredo Sauce.” But the new vendor only categorized it as “Sauce.” Having data analysts go through thousands of ingredients one by one was unrealistic. Technomic tried to create an internal rules based ingredient categorization system, but this approach proved inaccurate and time consuming. They contacted Lexalytics, who proposed a solution leveraging NLP and artificial intelligence (AI) to automatically and accurately categorize these ingredients.
SOLUTION
To test their approach, Lexalytics created a proof of concept in just two weeks for the “Sauces” category, which consisted of more than 2,300 different ingredient items. Using Technomic’s existing categories, Lexalytics trained a machine learning model to automatically put ingredients in the appropriate sub-categories under “Sauces.”
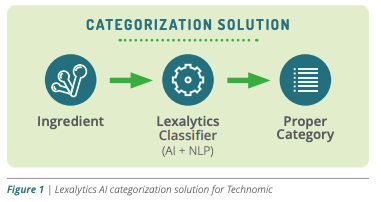
When a new ingredient comes into Technomic’s database, Lexalytics’ system automatically places it in the proper category. The system also assigns a “confidence range” of 0-100% that indicates its certainty that each ingredient is appropriately categorized. Given a confidence of 98%, for example, a data analyst can be certain that an ingredient is in the right bucket; whereas if the ingredient has a confidence of 20%, the analyst knows to double-check it.
RESULTS
After initial testing of the Lexalytics automatic categorization system, Technomic couldn’t be happier. Results show that Lexalytics placed ingredients into the proper categories 96.4% of the time. In fact, in 1.4% of instances an ingredient was found to be miscategorized by the Lexalytics model, further research showed that it was actually the result of human error. Lexalytics’ actual accuracy rate is 97.8%. This Lexalytics solution saves Technomic up to 40 people-hours of labor per category.
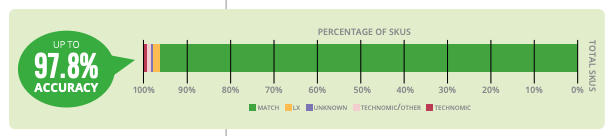
Figure 2 | Lexalytics AI categorization system for Technomic achieves up to 97.8% accuracy, saving up to 40 people-hours of labor per category, increasing category consistency, and freeing analysts to focus on customer-facing value-add analyses.
Lexalytics also enables Technomic to be more consistent in its category mapping by reducing the risk of human categorizers making dissimilar decisions. Additionally, the company is able to focus more on value-added analysis for its customers as opposed to back-end processes. As a result of the success of the pilot phase, Technomic has decided to use the Lexalytics system categorizing all 444,000+ of its ingredients moving forward.
Goals
- Determine whether a machine learning-based system for auto-categorization of hundreds of thousands of food ingredients is viable through a small-scale proof of concept
- Develop a system for automatically categorizing food ingredients based on their description
- Assign confidence level for categories to simplify the process of improving the system over time
Challenges
- Multiple data sources with different methods of categorization
- Vocabulary unique to food service
- Having multiple individuals apply category rules consistently
Solution
- Created an AI for Technomic that uses machine learning to automatically put ingredients in the proper categories based on product description
- Developed a confidence ranking to identify areas the model may need additional training
Results
- System properly categorized more than 2,300 ingredients in the “sauces” category 97.8% of the time
- Saved up to 40 hours of human coding work per category
- Will expand the project to extend across Technomic’s entire line of 444,000 ingredients and 375 categories